The Trouble with AI: There is More A than I
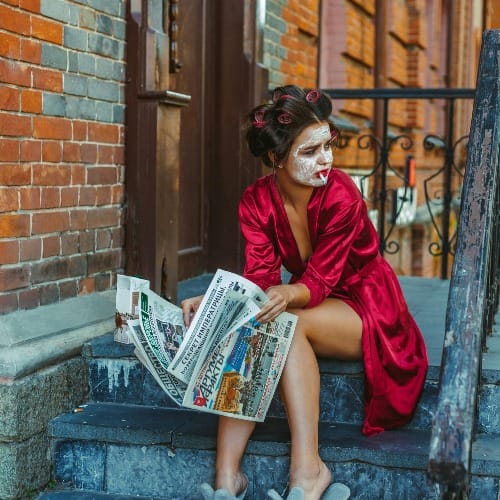
Lately, I wake up in the morning I step outside and say. WHAT'S GOING ON? For other Non-Blondes playing along at home...
How good are you at reading between the lines? I started laying out some information to keep what was going on straight. It's a little tricky. The post I linked to above is just the highlights. I won't over-interpret these events at this moment, but what screams out is that the controversy centers on not the tech itself but how it was built and the data used to train it. What data was scraped, how it was scraped, and how it was repackaged and used are all being vetted across multiple legal actions and executive orders.
Recalling that Altman's verbal defense as reported by the press was that he couldn't have done "it"--creating Chat GPT--without violating copyright. The LLM is not the innovation that Open AI introduced. Nor is semi-supervised learning nor NLP. Nor is pre-training. There is an alleged innovation in "scale and architecture," but this isn't easy to measure.
When you try to identify what was novel about GPT, the most salient thing is the size of the data set used in pre-training and the speed at which the training was done. Consequently, the most apparent "innovation" was how to scrape the entire internet and repackage it for sale with the alleged benefit of improving the quality of an LLM using GPT technology.
The perception of novelty depends on a lack of prior awareness of AI. For example, did you know our colleague David Cope has been creating AI-generated musical compositions since the 1990s? Or that writing tools such as Grammarly, Scribe, and Final Draft have used some form of AI and generative AI since the early oughts? I created an uproar on a consulting team by introducing the concept of generative UI software and telling UI designers to start upskilling in 2021. (I wonder if they have remembered me lately?)
Also, querying large databases for information is very old. I have a piece about Clippy, circa 1997, that allowed pretty decent queries of a knowledge management system concerning MS Office applications with rules-based AI. How far from Clippy is Chat GPT? It's parmetric and not rules based. It's not supervised learning but "semi-supervised" learning. Again, the main difference outside of rules-based versus parametric is scale.
If the innovation of contemporary LLMs is moving from supervised, rules-based learning to "parameters," we indeed have a more efficient and potentially flexible way to query large datasets. However, by combining enough parameters, as in billions, they function a little bit like rules. Because parameters make the rules implicit instead of explicit, we can call this "semi-supervised learning." Then, there is the issue of weighting using stochastic gradient descent and other Monte Carlo-like leveraging of randomness. If the weighting is in a black box, we can't be sure how much supervision is involved in the learning. "Fine-tuning" and supervision are distinctions with only a mild difference.
So again, the size and scale is novel: very little else. We now have a bunch of naked innovation emperors running around with measuring tapes.
I was initially persuaded that some advancement over prior technology existed because the speed and quality of results initially impressed us. However, further experimentation and reverse engineering revealed something was not adding up. We kept asking each other and ourselves, what is the true innovation of Chat GPT? While the power of the NLP querying was impressive and valuable. We haven't identified innovations that did not pre-exist in Chat GPT 1. It merely commanded a much more significant amount of backend data. We were at that time completely in the dark about what data was used and how it was obtained and we are still in the dark concerning the weighting, annotation, and normalization of that data. Something Musk's lawsuit specifically calls out.
I called it the Zillow-ification of AI. Zillow became what it is because it was the first to scrape publicly available real estate data and repackage it. The implications at the time were not apparent to me (although it may have been to others). I thought it clever. When they tried to topple the real estate agency business model, I worked in prop tech. There was a lot of pushback, however. Because that publicly owned data was only available to Zillow as citizens. When they made that data into a commercial package for sale, they became a different kind of actor. Before this, only brokerages that paid for access to the MLS could gather, store, and repackage such data for sale. They voluntarily stopped their iBuying business, however, do to revenue loss before any significant legal challenge could be brought.
Back to Altman's claim that he could not have built Chat GPT without violating copyright. The problem is that you don't need large volumes of data to create high-performing LLMs. In fact, there is a lot of evidence they work better with less data rather than more.
Why should we care about all of this in business? If my clients and yours or your organization invest in legally, federally, globally, and publicly contested technologies you are incurring risk. In that case, you will want to keep track of who the players are and what risks you incur in using these technologies and anticipate what legal and regulatory changes may dodge your investments before they get there.
What's between the lines here? It's too soon to say. Could the sequence of these events suggest collusion among multiple global actors to use AI not as an "intelligence" tool that can master larger reasoning tasks but as an intelligence tool, as in information valuable to those engaged in espionage, blackmail, and manipulating foreign affairs?
,